Once a business has successfully deployed its first AI feature to customers, the typical outcome is for Product teams to begin requesting more and more AI-powered functionality. Whilst good news for the business’ Data Scientists (who doesn’t like to be popular!), it can also pose AI/Data Science teams new challenges, particularly when AI features grow in complexity and require cross-functional team collaboration.
During our recent AI & Data Science Conference, we heard stories from several AI leaders in large B2B software companies on how they have successfully navigated the complexities of developing and deploying sophisticated AI-powered features at scale. One case study, Genesys, typifies this journey.
Building incremental AI-led features in companies with already-advanced AI products
At Genesys, a global cloud leader in customer experience orchestration, AI is a core component of the company’s solutions, Genesys Cloud CX and Genesys Multicloud CX, and the quality of which helps it to differentiate from the competition. With cutting edge AI algorithms either already in place or continually being created, the Genesys team is committed to consistent cross-functional team collaboration and strategic alignment on the product suite contributions.
Genesys has needed to evolve a way of working that facilitates rapid development, testing and deployment of AI features when the use case touches multiple business teams. Their approach has been to form project ‘squads’ (colleagues from different functions) to deliver AI features. Team members have a shared responsibility to develop and deploy AI features, with the business outcome (‘How does this improve the customer experience?’) always front of mind. Genesys has spent years perfecting their way of working in this respect; a business needs to find the right approach for their own culture, but the Genesys team shared the following top tips for others to consider:
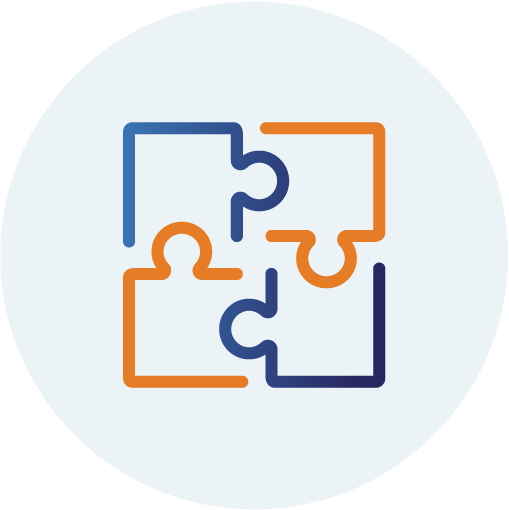 |
Plan Data Scientist and Software Engineer integration and collaboration from the start – not only when developing a new feature, but also when building a team. Avoid ‘ivory tower’ data science, and ensure that Data Scientists and Engineers co-develop with the business outcome and deployment requirements always front of mind. Failing AI projects are often caused by a disconnect between these two groups of resource.
|
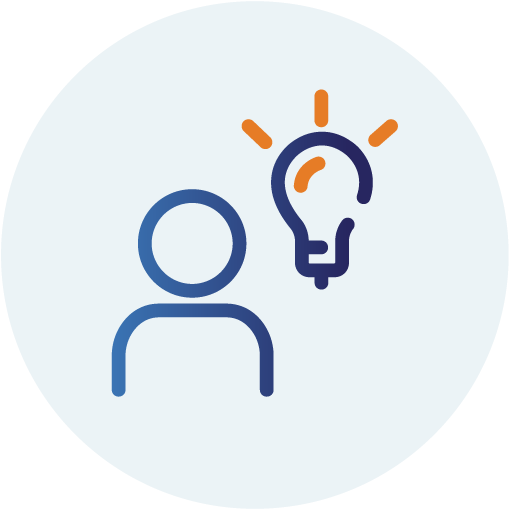 |
Identify ‘solution champions’ from the Product / Customer team at an early stage and bring them along the development journey – you can’t have too much feedback!
|
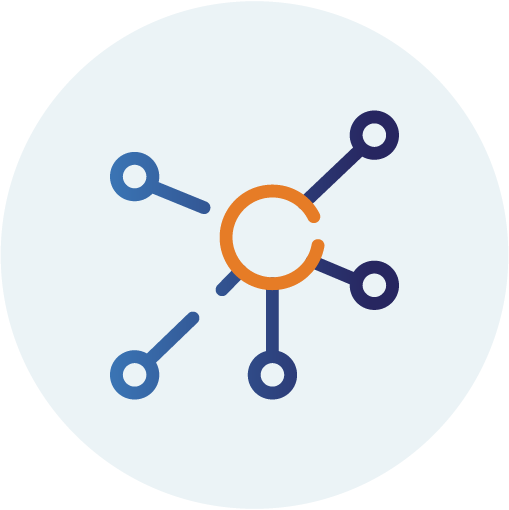 |
Never overlook the fact that ML code is only a small part of the overall AI/ML solution; the surrounding architecture is vast and complex and requires careful planning, adaptation and orchestration.
|