Data is a key organisational asset, however the path to applying Artificial Intelligence to data in a way that generates meaningful value can appear daunting and complex for B2B businesses. During Permira’s Data Science and AI Conference (4-6 May 2022), attended by technology leaders across 14 of our portfolio companies, we heard about three distinct ways in which B2B companies are successfully generating meaningful customer impact from AI capability:
- Empowering Internal Teams with AI-Driven Insight
- Launching AI Capability in Customer Products
- Scaling the Impact of AI Products
Across a series of three articles, we will share key insights from these discussions, drawing upon the real world experiences of our Portfolio community.
Empowering Internal Teams with AI-Driven Insight
Thanks to advancements in cloud-based technology, scalable storage and computation, and the growth of interoperable systems and open source tools - the ability to capture the rich data that businesses generate (customer-facing and internal interactions/events) has significantly improved over the past decade.
However, whilst data is becoming available in ever-increasing amounts, the ability to gather meaningful and actionable insights can be challenging. Organisations have had to build significant capability in areas such as data governance, transformation (from raw, unfiltered data into analysis-ready states), modelling, visualisation and embedding outputs into new and improved processes.
AI is recognised as a key capability area that can accelerate the ability to glean high-impact insights from large datasets and, depending on maturity of the organisation, embed in an automated way into existing processes and customer experience (often referred to as ‘prescriptive’ analytics).
Democratizing AI Insight Across Internal Teams
What does it mean to be a valued B2B technology solution or service provider? We heard that this is an area that has evolved significantly. Over the past 10 years, vendors have had to build considerably higher levels of customer intimacy across the lifecycle. Landing a customer and championing the features of a product is no longer sufficient. Instead, a much higher level of coordinated, ‘customer obsession’ is required from sales, account management and customer success teams to ensure customers are actually realising value from the product. This implies the need to become more responsive to changes in customers’ requirements, navigating commercial and organisational imperatives and demonstrating true partnership to help customers become successful. Getting this right creates loyalty and helps maximise Customer Lifetime Value (CLV).
Throughout the Data Science and AI Conference, we heard about how AI capability is helping internal teams (e.g., Customer Success, Sales, Renewals) know their customers better than ever, make better decisions on how to serve them and support faster action-taking. The impact? Renewal rates, up/cross-sell rates and NPS scores all soared.
Informatica, an enterprise cloud data management provider, have built an internal AI-powered platform called ‘Cassini’ that is used by Customer Success, Support, Sales and Renewal teams. It helps them proactively engage customers, mitigate churn, personalize the renewals process, and auto-recommend product that best suit a customer’s needs as they evolve (maximising upsell and cross-sell revenue whilst appearing a ‘helpful’ and ‘in tune’ vendor). Key learnings from the Informatica journey include:
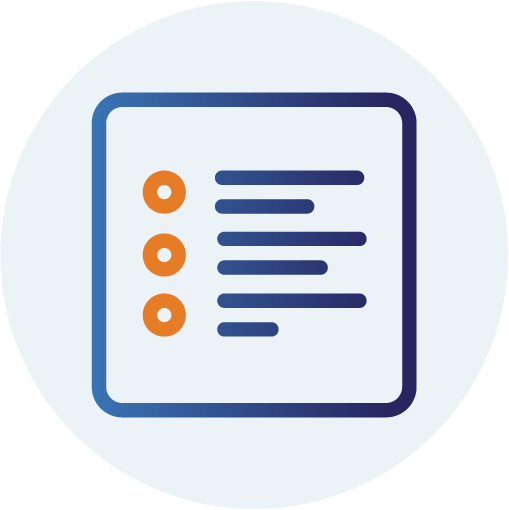 |
Solve for use cases: start with one, prove value and then move onto the next (sourcing and cleaning data only as the use case requires)
|
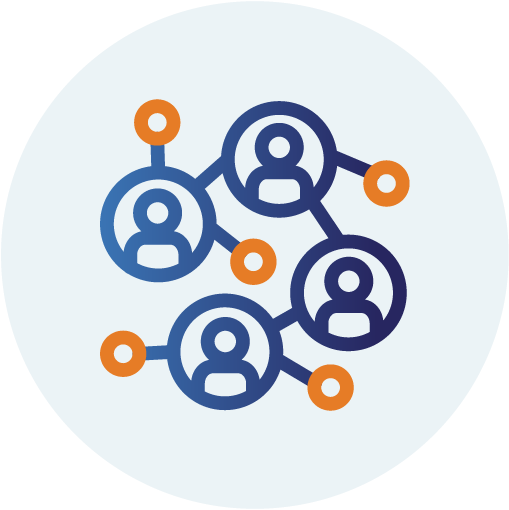 |
Collaborate with your internal users as early and as much as possible: they are your customers, their feedback is critical to the Data Science team
|
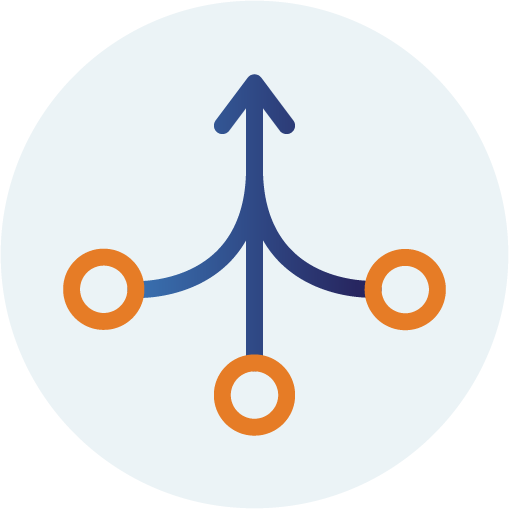 |
UI matters: present AI insight to internal teams as cleanly, crisply and with as little ‘cognitive overhead’ as possible via curated data (that answers specific questions) and visual outputs. Wherever possible integrate deeply into existing workflows and systems
|
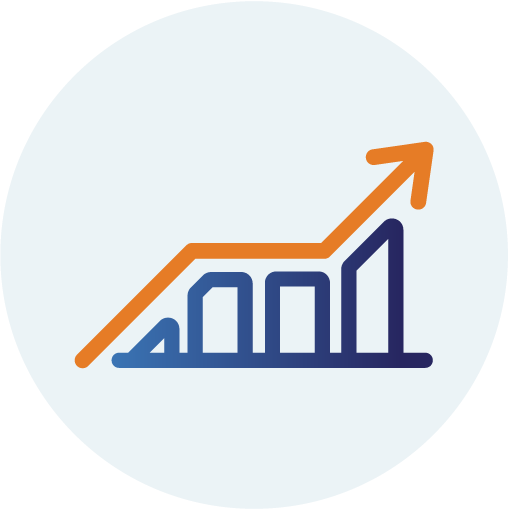 |
Track and measure outcomes: define the KPIs you are looking to influence, and ensure robust measurement is in place to demonstrate impact (progress is more important than perfection!)
|
With increasing accessibility and quality of cloud-based data lakes and virtualised data warehouses, we expect the barriers to B2B companies to develop AI-based capability to support internal teams to increasingly lower. The most successful companies will be those that take a disciplined approach to defining and identifying valuable use cases, and which can foster a cross-team culture of collaboration between functional users and Data Scientists to ensure AI capability translates into meaningful insight that can be embedded into user workflows.