Many B2B software providers are looking to enhance their customer experiences with AI-powered features and functionality. During our AI and Data Science Conference, we heard that taking the first step – taking that first AI feature from idea to production – can be daunting and challenging. Yet the rewards can be sizeable, and the challenges navigable with a disciplined approach.
Technology leaders from across our Portfolio were keen to point out that having a clear goal, the right expectations from outset and an incremental, stepwise strategy (rather than expecting ‘big bang’ gains from AI) is paramount for deploying AI successfully in software products. This was illuminated in a case study of Nexthink, an employee experience management platform.
Start simple and build incremental AI functionality over time
Nexthink’s software platform contains several AI-driven features today, however the journey to get to this point generated many lessons learned, which were discussed during our Conference. From a standing start, Nexthink have developed a suite of AI-based models that identify root causes of IT issues that constrain employee productivity and recommend remediation steps. Nexthink’s Head of Data Science, boiled the business’ successful journey to scaled AI deployment down to three core concepts:
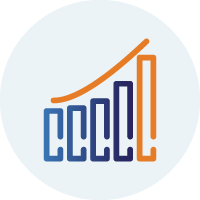 |
Start small;
Grow capability incrementally, one use case at a time
|
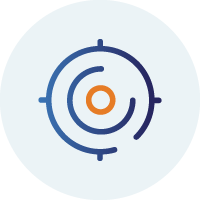 |
Be hyper-targeted;
Use AI sparingly and only where it has a clear, measurable value-add. A common mistake is to solve a problem which is not aligned to business priorities
|
 |
Maintain explainability;
Simple usually trumps complex, as ultimately business stakeholders need to trust the outputs. Another common mistake is to deploy a complex AI algorithm when a simpler one would meet business needs.
|
Case in point, Nexthink’s first production release of a model for automatic IT troubleshooting was a simple, easy-to-explain statistical model. Internal product owners loved it and it could be moved to production swiftly – customers began getting immediate benefit with an improved experience of faster issue resolution. The team then enhanced the model using more advanced techniques – version 2.0 leveraged a Bayesian approach. The team is currently working on version 3.0, a sophisticated technique known as Causal Inference. This can enable automatic troubleshooting by combining real-time inference on the causal relationships between changes in a company’s IT environment and a user’s issues/problems with statistical analyses of the impact of possible remediation actions (e.g. Average Treatment Effect computation).
Nexthink’s Data Scientists continue to make the effort to unpack the algorithm into explainable factors that can be communicated to business stakeholders – they remind us that typically a significant amount of value comes from a simple, baseline model that does not require training! AI models are complex to build and tune; it often takes time to develop levels of explain-ability that customers, product teams and support teams will be able to interpret and act on. As such starting simple, focusing on customer impact and increasing levels of complexity over time is a critical ingredient of success.